The Ultimate Guide to Semantic Segmentation Labeling Tools
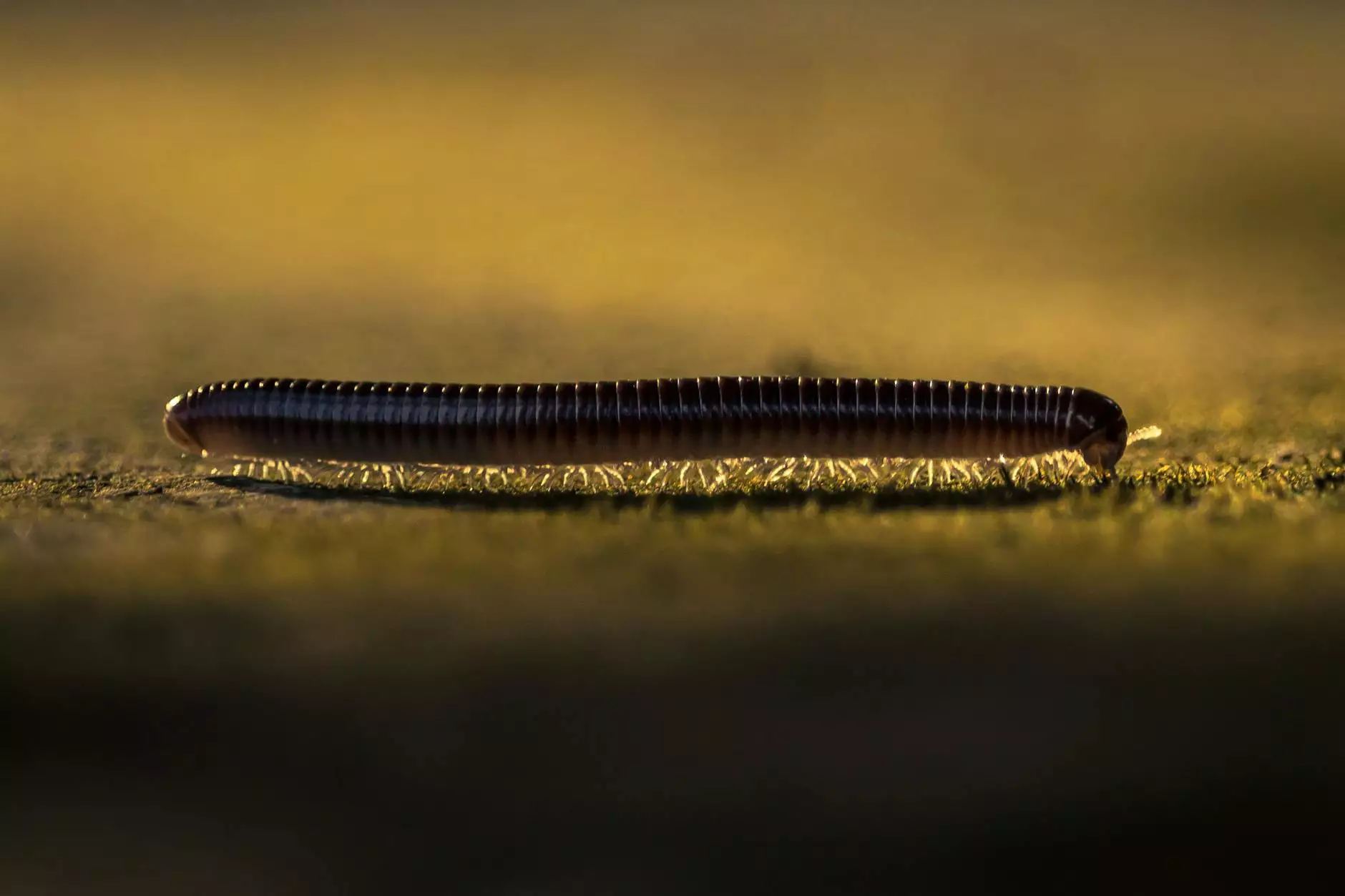
In the world of artificial intelligence (AI) and machine learning (ML), data is king. The foundation of machine learning models rests upon the quality and richness of the data used to train them. Among various data types, images stand out due to their complexity and the wealth of information they encode. To harness this potential effectively, businesses frequently turn to semantic segmentation labeling tools.
Understanding Semantic Segmentation
Semantic segmentation is a process where an image is divided into segments, each corresponding to a meaningful category. Unlike standard image classification, where the goal is to identify the primary object in an image, semantic segmentation classifies every pixel in an image. This granular labeling allows for a comprehensive understanding of the scene, useful in advanced applications such as autonomous vehicles, medical imaging, and object detection.
The Importance of Data Annotation
Data annotation is essential in developing machine learning models. High-quality annotated data leads to better model performance, accuracy, and validity. Businesses rely on various data annotation techniques, including bounding box, polygon, and semantic segmentation, to ensure they have the right data for training.
Why Choose Semantic Segmentation?
- Detailed Understanding: Segmentation offers a detailed understanding of each object and its context within an image.
- Enhanced Training: Models trained on segmented data can better generalize their understanding of different objects.
- Usefulness in a Variety of Industries: From healthcare to agriculture, segments provide tailored insights that bolster operational efficiency.
Features of an Effective Semantic Segmentation Labeling Tool
Choosing the right semantic segmentation labeling tool is crucial for the success of any AI model. The following features should be considered:
1. User-Friendly Interface
A tool with an intuitive interface minimizes the learning curve for users and enhances productivity. User experience should always be prioritized to facilitate smooth project workflows.
2. Scalability
As businesses grow, so do their data annotation needs. A good semantic segmentation labeling tool should be able to handle an increasing volume of data without sacrificing performance.
3. Integration Capabilities
In today’s tech ecosystem, seamless integration with existing software and workflows is crucial. Look for tools that provide APIs and plugins for easy data transfers and embedding into existing systems.
4. Collaboration Features
In many businesses, data annotation is a team effort. Tools that support collaboration allow multiple annotators to work together, improving efficiency and consistency in labeling efforts.
5. Quality Control Mechanisms
Ensuring data quality is non-negotiable in machine learning models. Tools that offer built-in quality assurance, validation checks, and revision workflows help maintain high standards of data integrity.
Benefits of Using Keylabs’ Semantic Segmentation Labeling Tool
When it comes to data annotation tools, Keylabs.ai stands out for several reasons:
Comprehensive Solution
Keylabs offers an all-in-one platform that encompasses a variety of annotation methods, with a particular strength in semantic segmentation. This versatility enables businesses to efficiently annotate diverse datasets without switching tools.
Advanced AI-Assisted Features
Our platform incorporates AI-driven capabilities that expedite the annotation process. Smart algorithms assist in predicting segments, reducing manual work, and allowing human annotators to focus on complex tasks.
Real-Time Collaboration
With Keylabs, teams can work together in real-time, ensuring that all contributors are aligned and that feedback is instantaneous. This feature inherently boosts productivity and project timelines.
Robust Support and Documentation
Keylabs.ai understands the importance of customer support. Our team provides comprehensive documentation and responsive customer service, making it easy for users to find solutions swiftly to any issues they may encounter.
How to Implement a Semantic Segmentation Labeling Tool
Successful implementation of a semantic segmentation labeling tool involves several essential steps:
1. Define Your Objectives
Before selecting a tool, clearly define what you aim to achieve with semantic segmentation. Different projects may require particular features or specifications.
2. Choose the Right Tool
Based on your objectives, evaluate various tools available in the market. Consider features such as ease of use, integration, and scalability, especially focusing on tools like Keylabs.ai that excel in these areas.
3. Train Your Team
Once you have selected a tool, invest time in training your team to ensure they are proficient in using it. This will minimize errors and optimize the annotation process.
4. Start Annotating
Begin the annotation process, starting with a pilot project. This will allow you to identify any issues in your workflow and refine processes before scaling up.
5. Continuous Improvement
Regularly review the quality of your annotated data and seek feedback from users. Continuously iterating on techniques and workflows will greatly improve the overall efficiency of your data annotation efforts.
Common Challenges in Data Annotation
While semantic segmentation labeling tools offer immense benefits, businesses may encounter challenges, including:
- Data Quality: Poor-quality data can hinder the effectiveness of ML models.
- Annotator Bias: Individual biases in annotation can create inconsistencies.
- Time Consumption: Manual annotation can be time-consuming without the right tools in place.
Tackling Annotation Challenges with Keylabs
Keylabs.ai not only provides semantic segmentation labeling tools but also incorporates machine learning features designed to mitigate common annotation challenges:
Automating Repetitive Tasks
By automating mundane segmentation tasks, our platform allows you to focus on the more critical aspects of data preparation, thus saving time and reducing errors.
Ensuring Consistency Through Guidelines
Keylabs.ai provides features that indicate and enforce annotation guidelines, ensuring consistency across the team regardless of the number of annotators and their experience levels.
Conclusion
As the demand for high-quality data continues to escalate, the importance of semantic segmentation labeling tools cannot be overstated. Keylabs.ai uniquely positions itself as a leading solution in the market, catering to the diverse annotation needs of businesses across multiple sectors. Investing in a semantic segmentation tool will not merely enhance your data's quality; it will pay dividends in the form of superior AI models, resulting in improved business outcomes and operational efficiency.
By harnessing the full capabilities of a robust semantic segmentation labeling tool like Keylabs.ai, you set the stage for success in your data-driven ventures.
Explore more about our services at keylabs.ai.